Keynote
Accelerating RF Autonomy for Uncertain Environments
Under the Radiofrequency (RF) Machine Learning Systems (RFMLS) program, DARPA developed foundational technologies that enable autonomous RF systems to learn directly from data. By applying deep neural networks (DNNs) directly to digitized RF signals, RFMLS extracted more discriminating signal features and accelerated the implementation of detectors relative to traditional signal processing approaches.
RFMLS demonstrated the value of attentional algorithms that focus on important signals. Extending this concept of attention to emerging runtime reconfigurable processors may enable edge systems to process higher signal densities across wider bandwidths.
RFMLS focused on supervised learning across labelled datasets, but these approaches have reduced applicability when systems must operate in highly uncertain environments. Sample efficient learning algorithms coupled with automated design of experiences has the potential to improve the robustness of autonomous RF systems that must operate in environments that are difficult to specify at design time.
This talk will further discuss key results of the RFMLS program, open related technical challenges, and corresponding potential areas for future research.
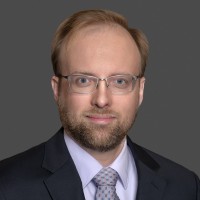
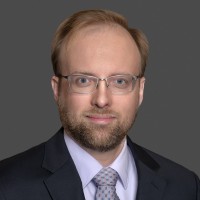
John Davies
John Davies is a program manager in DARPA’s Microsystems Technology Office (MTO). His research interests include adaptive radio frequency (RF) systems, artificial intelligence, real-time signal processing, and configurable computing. Prior to joining DARPA in 2020, Mr. Davies was a branch director and technical fellow with the Leidos Innovation Center. While at Leidos, he oversaw a portfolio of programs that applied artificial intelligence to real-time RF systems. Prior to Leidos, Mr. Davies was a technical development manager for BAE Systems where he led multiple R&D efforts focused on cognitive communications, RF sensing, and real-time signal processing. Mr. Davies earned his Bachelor of Science and Master of Science degrees in electrical engineering from Virginia Tech.